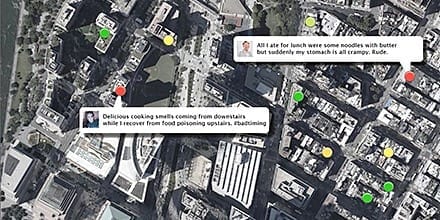
It’s happened to many of us. We eat at a restaurant with less than ideal hygiene and come down with a nasty case of food poisoning.
Foodborne illness afflicts 48 million people annually in the U.S. alone; 120,000 individuals are hospitalized annually, and 3,000 die from the illness. In fact, one out of every six Americans gets food poisoning each year. And many of these sufferers write about it on Twitter.
Computer science researchers from the University of Rochester have developed an app for health departments that uses natural language processing and artificial intelligence to identify food poisoning-related tweets, connect them to restaurants using geotagging and identify likely hot spots.
The team presented the results of its research at the 30th Association for the Advancement of Artificial Intelligence (AAAI) conference in Phoenix, AZ, in February. The project was supported by grants from the National Science Foundation (NSF), the National Institutes of Health and the Intel Science and Technology Center for Pervasive Computing.
Location-based epidemiology is nothing new. John Snow, credited as the world’s first epidemiologist, used maps of London in 1666 to identify the source of the Cholera epidemic that was rampaging the city (a neighborhood well) and in the process discovered the connection between the disease and water sources.
However, as the researchers showed, it’s now possible to deduce the source of outbreaks using publicly available social media content and deep learning algorithms trained to recognize the linguistic traits associated with a disease — “I feel nauseous,” for instance.
Learn more: nEmesis Fights Foodborne Illnesses with Machine Learning
The Latest on: Machine learning
[google_news title=”” keyword=”machine learning” num_posts=”10″ blurb_length=”0″ show_thumb=”left”]
via Google News
The Latest on: Machine learning
- New machine learning algorithm promises advances in computingon May 9, 2024 at 3:03 pm
Systems controlled by next-generation computing algorithms could give rise to better and more efficient machine learning products, a new study suggests.
- Machine learning tool flags undiagnosed immunodeficiency diseaseon May 9, 2024 at 6:00 am
A machine learning model can effectively sift through EHR data to rank patients' likelihood of having common variable immunodeficiency disease.
- Five Takeaways: Artificial Intelligence & Machine Learningon May 9, 2024 at 12:00 am
The Journal of Business hosted Yanek Kondryszyn, intellectual property attorney and partner at Lee & Hayes PLLC, for its most recent Elevating The Conversation podcast.
- Machine Learning Takes the Stage at TC Sessions: Robotics+AIon May 8, 2024 at 2:00 pm
The roots of machine learning go back at least to 1962, when Doug Engelbart of the Stanford Research Institute argued that computers could play a role in augmenting the human intellect. Outlandish at ...
- Machine learning aids in discovery of sperm whale ‘alphabet’on May 8, 2024 at 11:01 am
Researchers at MIT CSAIL and Project CETI believe that they have unlocked a kind of sperm whale alphabet with the aid of machine learning technologies.
- The Future Of SEO: How AI And Machine Learning Are Changing Searchon May 8, 2024 at 4:45 am
Despite the uncertainty about how search engines adjust to the new AI world, machine learning is revolutionizing—for the better—the way businesses approach SEO.
- From Beginner To Enthusiast: Top 5 Free Harvard Courses To Spark Your AI Journey With Machine Learning, Python And Moreon May 8, 2024 at 3:55 am
Ever thought about diving into the world of Artificial Intelligence (AI) but felt intimidated by the hefty price tags on courses? Well, Harvard University is offering a golden opportunity to get ...
- Machine learning helps to discover sperm whale ‘alphabet'on May 8, 2024 at 3:07 am
Researchers have cracked the code to a sperm whale ‘alphabet' after using machine learning technologies to understand vocalizations. In a… Continue reading Machine learning helps to discover sperm ...
- Machine learning engineer salary: How much do AI techs make?on May 7, 2024 at 4:02 pm
Machine learning engineers tend to be among the highest-paid employees in tech. Here’s how much they make and which companies pay the most.
- Tomorrow’s physics test: machine learningon May 7, 2024 at 7:20 am
Machine learning is becoming an essential part of a physicist’s toolkit. How should new students learn to use it?
via Bing News