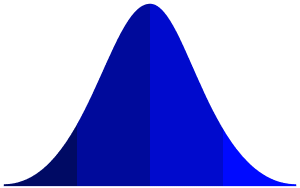
Imagine if there were a simple single statistical measure everybody could use with any set of data and it would reliably separate true from false. Oh, the things we would know! Unrealistic to expect such wizardry though, huh?
Yet, statistical significance is commonly treated as though it is that magic wand. Take a null hypothesis or look for any association between factors in a data set and abracadabra! Get a “p value” over or under 0.05 and you can be 95% certain it’s either a fluke or it isn’t. You can eliminate the play of chance! You can separate the signal from the noise!
Except that you can’t. That’s not really what testing for statistical significance does. And therein lies the rub.
Testing for statistical significance estimates the probability of getting roughly that result if the underlying hypothesis is assumed to be true. It can’t on its own tell you whether this assumption was right, or whether the results would hold true in different circumstances. It provides a limited picture of probability, because it takes limited information about the data into account.
What’s more, the finding of statistical significance itself can be a “fluke,” and that becomes more likely in bigger data and when you run the test on multiple comparisons in the same data. You can read more about that here.
Statistical significance testing can easily sound as though it sorts the wheat from the chaff, but it’s not enough to do that on its own – and it can break down in the face of many challenges. Nor do all tests of statistical significance work the same way on all data sets. And what’s more, “significant” doesn’t mean it’s important either. A sliver of an effect can reach the less-than-5% threshold. We’ll come back to what all this means practically shortly.
The common approach to statistical significance testing was so simple to grasp, though, and so easy to do even before there were computers, that it took the science world by storm. As Stephen Stigler explains in his piece on Fisher and the 5% level, “it opened the arcane domain of statistical calculation to a world of experimenters and research workers.”
But it also led to something of an avalanche of abuses. The over-simplistic approach to statistical significance has a lot for which to answer. As John Ioannidis points out here, this is a serious player in science’s failure to replicate results.
Go deeper with Bing News on:
Statistical significance
- El Paso School District reports significant decrease in chronic student absences
The El Paso Independent School District said they've gotten more of their students back in the classrooms. The statistics shared with KFOX14 show a decrease in the number of "chronically absent ...
- Tarsus Reports First Quarter 2024 Financial Results and Recent Business Achievements
Both trials met the primary endpoint and all secondary endpoints, with statistical significance and no serious treatment-related adverse events. Most patients found the XDEMVY eye drop to be neutral ...
- Vitamin D receptor polymorphism found to influence COVID-19 severity
VDR gene polymorphisms affecting VDR function are associated with immune responses. In COVID-19 patients, various VDR polymorphisms correlate with different symptoms, such as shortness of breath, ...
- Employee benefits statistics in 2024
Mercer figures suggest that parental leave policies are becoming more popular and are broadening to be more inclusive. The number of employers offering paid parental leave grew to 75% in 2023 ...
- Statistical analysis highlights the benefits of Mediterranean Diet on emotional well-being
The relationship between adherence to the Mediterranean Diet (MD), subjective well-being (SWB), and various socioeconomic factors ...
Go deeper with Google Headlines on:
Statistical significance
[google_news title=”” keyword=”Statistical significance” num_posts=”5″ blurb_length=”0″ show_thumb=”left”]
Go deeper with Bing News on:
Statistical hypothesis testing
- How to Critically Appraise an Article
Other factors that also should be considered include the suitability of the statistical methods used and their subsequent interpretation, potential conflicts of interest and the relevance of the ...
- Workshop on SPSS concludes at Srinath University Jamshedpur
An Introduction,’ hosted by the School of Commerce and Management at Srinath University, concluded on Saturday. Attended by nearly 150 participants from esteemed institutions across the region, the ...
- The Cookie Test Helps Decide Public College Or Private College
Amidst college admissions noise, the Cookie Test helps students determine if they will succeed in a public college or if the would be better served at a private college.
- New Manuscripts
NEJM uses highly rigorous editorial, peer, and statistical review processes to evaluate manuscripts for scientific accuracy, novelty, and importance. Step 2: Prepare materials for submission ...
- Theoretical biologists test two modes of social reasoning and find surprising truths in simplicity
Imagine a small village where every action someone takes, good or bad, is quietly followed by ever-attentive, nosy neighbors. An individual's reputation is built through these actions and observations ...
Go deeper with Google Headlines on:
Statistical hypothesis testing
[google_news title=”” keyword=”Statistical hypothesis testing” num_posts=”5″ blurb_length=”0″ show_thumb=”left”]