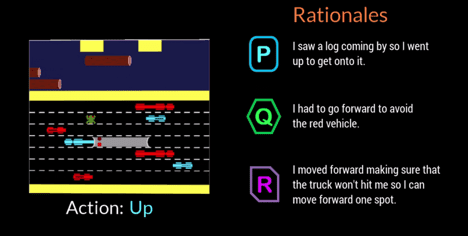
Why did the frog cross the road? Well, a new artificial intelligent (AI) agent that can play the classic arcade game Frogger not only can tell you why it crossed the road, but it can justify its every move in everyday language.
Developed by Georgia Tech, in collaboration with Cornell and the University of Kentucky, the work enables an AI agent to provide a rationale for a mistake or errant behavior, and to explain it in a way that is easy for non-experts to understand.
This, the researchers say, may help robots and other types of AI agents seem more relatable and trustworthy to humans. They also say their findings are an important step toward a more transparent, human-centered AI design that understands people’s preferences and prioritizes people’s needs.
“If the power of AI is to be democratized, it needs to be accessible to anyone regardless of their technical abilities,” said Upol Ehsan, Ph.D. student in the School of Interactive Computing at Georgia Tech and lead researcher.
“As AI pervades all aspects of our lives, there is a distinct need for human-centered AI design that makes black-boxed AI systems explainable to everyday users. Our work takes a formative step toward understanding the role of language-based explanations and how humans perceive them.”
The study was supported by the Office of Naval Research (ONR).
Researchers developed a participant study to determine if their AI agent could offer rationales that mimicked human responses. Spectators watched the AI agent play the videogame Frogger and then ranked three on-screen rationales in order of how well each described the AI’s game move.
Of the three anonymized justifications for each move – a human-generated response, the AI-agent response, and a randomly generated response – the participants preferred the human-generated rationales first, but the AI-generated responses were a close second.
Frogger offered the researchers the chance to train an AI in a “sequential decision-making environment,” which is a significant research challenge because decisions that the agent has already made influence future decisions. Therefore, explaining the chain of reasoning to experts is difficult, and even more so when communicating with non-experts, according to researchers.
In case the study participants weren’t familiar, the game’s goal of getting the frog safely home without being hit by moving vehicles or drowned in the river was explained to them. The simple game mechanics of moving up, down, left or right, allowed the participants to see what the AI was doing, and to reasonably evaluate if the rationales on the screen clearly justified the move.
The participants judged the rationales based on:
- Confidence – the person is confident in the AI to perform its task
- Human-likeness – looks like it was made by a human
- Adequate justification – adequately justifies the action taken
- Understandability – helps the person understand the AI’s behavior
AI-generated rationales that were ranked higher by participants were those that showed recognition of environmental conditions and adaptability, as well as those that communicated awareness of upcoming dangers and planned for them. Redundant information that just stated the obvious or misrepresented the environment were found to have a negative impact.
“This project is more about understanding human perceptions and preferences of these AI systems than it is about building new technologies,” said Ehsan. “At the heart of explainability is sensemaking. We are trying to understand that human factor.”
A second related study validated the researchers’ decision to design their AI agent to be able to offer one of two distinct types of rationales:
- Concise, “focused” rationales or
- Holistic, “complete picture” rationales
In this second study, participants were only offered AI-generated rationales after watching the AI play Frogger. They were asked to select the answer that they preferred in a scenario where an AI made a mistake or behaved unexpectedly. They did not know the rationales were grouped into the two categories.
By a 3-to-1 margin, participants favored answers that were classified in the “complete picture” category. Responses showed that people appreciated the AI thinking about future steps rather than just what was in the moment, which might make them more prone to making another mistake. People also wanted to know more so that they might directly help the AI fix the errant behavior.
“The situated understanding of the perceptions and preferences of people working with AI machines give us a powerful set of actionable insights that can help us design better human-centered, rationale-generating, autonomous agents,” said Mark Riedl, professor of Interactive Computing and lead faculty member on the project.
A possible future direction for the research will apply the findings to autonomous agents of various types, such as companion agents, and how they might respond based on the task at hand. Researchers will also look at how agents might respond in different scenarios, such as during an emergency response or when aiding teachers in the classroom.
Learn more: Research Findings May Lead to More Explainable AI
The Latest on: AI agents
[google_news title=”” keyword=”AI agents” num_posts=”10″ blurb_length=”0″ show_thumb=”left”]
via Google News
The Latest on: AI agents
- Agents of manipulation (the real AI risk)on May 17, 2024 at 7:05 pm
Artificial agents will make our lives better. At the same time, these superpowers could easily be deployed as agents of manipulation.
- AI marketplace connects robot agents to pay humans to complete jobson May 16, 2024 at 9:29 pm
Payman is a marketplace that was created for AI robots to pay humans who can get do a job they can't. NBC News' Gadi Schwartz talks to Nita A. Farahany about the marketplace and the future of jobs in ...
- Protocol Village: FLock.io to Merge Decentralized AI Training With Morpheus 'Smart Agents'on May 16, 2024 at 3:03 pm
FLock.io, a platform for "AI co-creation," and Morpheus, a network for powering smart agents, announced a strategic partnership "to advance decentralized AI capabilities in Web3," according to the ...
- An AI agent may soon be at your beck and call, but it might cost you a bit of your privacyon May 15, 2024 at 5:52 am
The stakes for Google are high, as nailing AI agents opens up a massive business opportunity.
- Google takes on GPT-4o with Project Astra, an AI agent that understands dynamics of the worldon May 14, 2024 at 8:58 pm
Google says it plans to add some Project Astra capabilities to the Gemini mobile and web experiences later this year.
- Everything announced at Google I/O, including AI Agents, Ask Photos, and moreon May 14, 2024 at 12:16 pm
Google announced at I/O that Google Photos would get a powerful new AI tool called Ask Photos. Google said the feature can effectively parse through your pictures and answer questions you might have, ...
- Google strikes back at OpenAI with “Project Astra” AI agent prototypeon May 14, 2024 at 12:11 pm
Google also announced a new AI model called Gemini 1.5 Flash, which it billed as a lightweight, faster, and less expensive version of Gemini 1.5. "1.5 Flash is the newest addition to the Gemini model ...
- Google just gave us a tantalizing glimpse into the future of AI agentson May 14, 2024 at 10:51 am
Google is trying to turn AI chatbots into intelligent systems that can reason, plan, and remember. Project Astra is a window into this future.
- Google I/O 2024: 'AI Agents' are AI personal assistants that can return your shoeson May 14, 2024 at 10:51 am
Google will be sharing lots of products ready to utilize at today's big Google I/O event. However, one announcement from CEO Sundar Pichai was more of an idea that's in the works: AI Agents. According ...
- Retell AI lets companies build 'voice agents' to answer phone callson May 9, 2024 at 6:30 am
Call centers are embracing automation. According to research firm TechSci Research, the global market for contact center AI could grow to nearly $3 billion in 2028, from $2.4 billion in 2022.
via Bing News