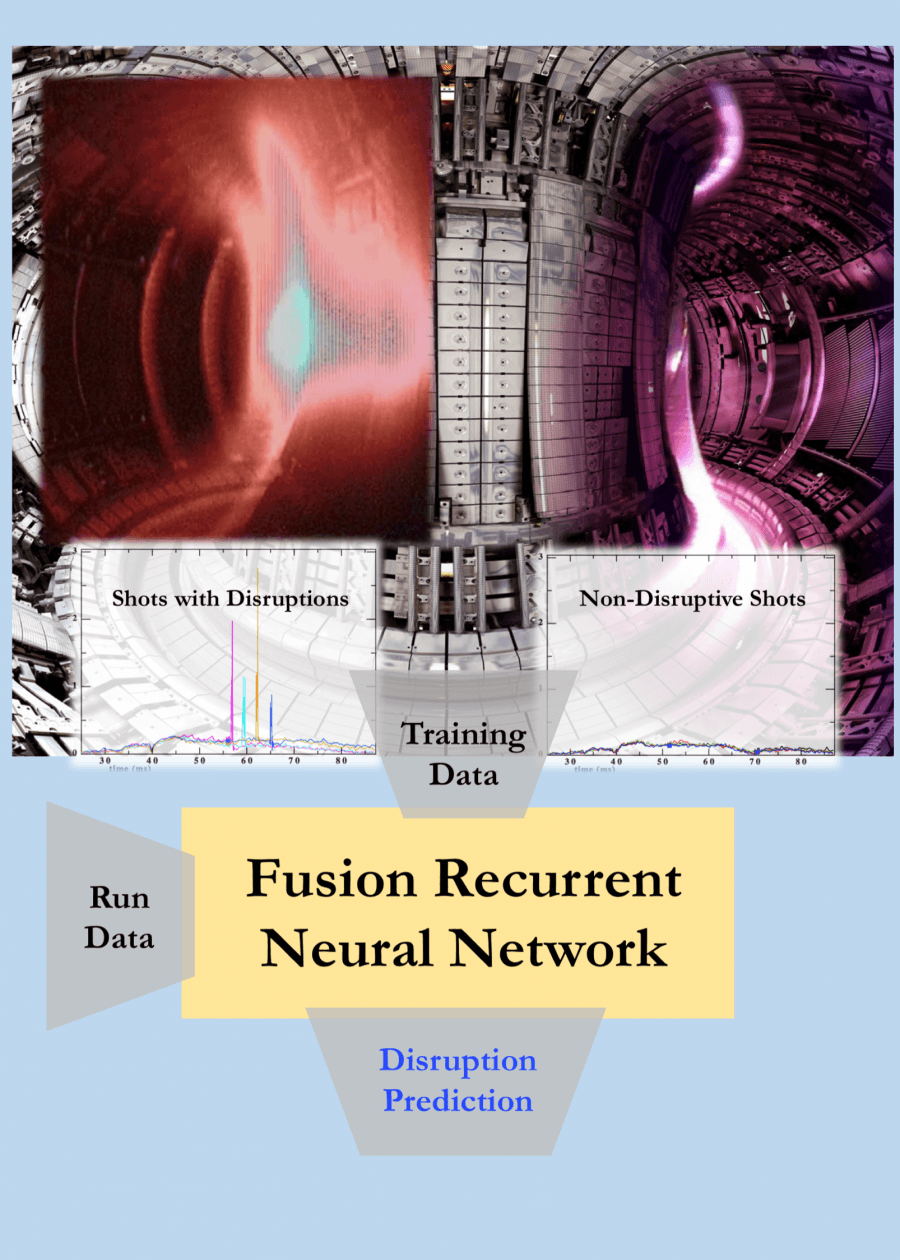
Before scientists can effectively capture and deploy fusion energy, they must learn to predict major disruptions that can halt fusion reactions and damage the walls of doughnut-shaped fusion devices called tokamaks. Timely prediction of disruptions, the sudden loss of control of the hot, charged plasma that fuels the reactions, will be vital to triggering steps to avoid or mitigate such large-scale events.
Today, researchers at the U.S. Department of Energy’s (DOE) Princeton Plasma Physics Laboratory (PPPL) and Princeton University are employing artificial intelligence to improve predictive capability. Researchers led by William Tang, a PPPL physicist and a lecturer with the rank and title of professor at Princeton University, are developing the code for predictions for ITER, the international experiment under construction in France to demonstrate the practicality of fusion energy.
Form of “deep learning”
The new predictive software, called the Fusion Recurrent Neural Network (FRNN) code, is a form of “deep learning” — a newer and more powerful version of modern machine- learning software, an application of artificial intelligence. “Deep learning represents an exciting new avenue toward the prediction of disruptions,” Tang said. “This capability can now handle multi-dimensional data.”
FRNN is a deep-learning architecture that has proven to be the best way to analyze sequential data with long-range patterns. Members of the PPPL and Princeton University machine-learning team are the first to systematically apply a deep learning approach to the problem of disruption forecasting in tokamak fusion plasmas.
Chief architect of FRNN is Julian Kates-Harbeck, a graduate student at Harvard University and a DOE-Office of Science Computational Science Graduate Fellow. Drawing upon expertise gained while earning a master’s degree in computer science at Stanford University, he has led the building of the FRNN software.
More accurate predictions
Using this approach, the team has demonstrated the ability to predict disruptive events more accurately than previous methods have done. By drawing from the huge data base at the Joint European Torus (JET) facility located in the United Kingdom — the largest and most powerful tokamak in operation — the researchers have significantly improved upon predictions of disruptions and reduced the number of false positive alarms. EUROfusion, the European Consortium for the Development of Fusion Energy, manages JET research.
The team now aims to reach the challenging goals that ITER will require. These include producing 95 percent correct predictions when disruptions occur, while providing fewer than 3 percent false alarms when there are no disruptions. “On the test data sets examined, the FRNN has improved the curve for predicting true positives while reducing false positives,” said Eliot Feibush, a computational scientist at PPPL, referring to what is called the “Receiver Operating Characteristic” curve that is commonly used to measure machine learning accuracy. “We are working on bringing in more training data to do even better.”
Highly demanding
The process is highly demanding. “Training deep neural networks is a computationally intensive task that requires engagement of high-performance computing hardware,” said Alexey Svyatkovskiy, a Princeton University big data researcher. “That is why a large part of what we do is developing and distributing new algorithms across many processors to achieve highly efficient parallel computing. Such computing will handle the increasing size of problems drawn from the disruption-relevant data base from JET and other tokamaks.”
The deep learning code runs on graphic processing units (GPUs) that can compute thousands of copies of a program at once, far more than older central processing units (CPUs). Tests performed on modern GPU clusters, and on world-class machines such as Titan, currently the fastest and most powerful U.S. supercomputer at the Oak Ridge Leadership Computing Facility, a DOE Office of Science User Facility at Oak Ridge National Laboratory, have demonstrated excellent linear scaling. Such scaling reduces the computational run time in direct proportion to the number of GPUs used — a major requirement for efficient parallel processing.
Princeton’s Tiger cluster
Princeton University’s Tiger cluster of modern GPUs was the first to conduct deep learning tests, using FRNN to demonstrate the improved ability to predict fusion disruptions. The code has since run on Titan and other leading supercomputing GPU clusters in the United States, Europe and Asia, and have continued to show excellent scaling with the number of GPUs engaged.
Going forward, the researchers seek to demonstrate that this powerful predictive software can run on tokamaks around the world and eventually on ITER. Also planned is enhancement of the speed of disruption analysis for the increasing problem sizes associated with the larger data sets prior to the onset of a disruptive event. Support for this project has primarily come to date from the Laboratory Directed Research and Development funds provided by PPPL.
Learn more: Artificial intelligence helps accelerate progress toward efficient fusion reactions
The Latest on: Fusion Recurrent Neural Network
[google_news title=”” keyword=”Fusion Recurrent Neural Network” num_posts=”10″ blurb_length=”0″ show_thumb=”left”]- Unlocking consciousness: A new frontier in neuroscientific fusionon May 8, 2024 at 1:08 pm
Dr. Marx and Prof. Gilon propose that memory plays a pivotal role in shaping consciousness, contrasting the idea that computer-based Information Theory provides a sufficient framework for ...
- Drift of neural ensembles driven by slow fluctuations of intrinsic excitabilityon May 7, 2024 at 5:33 am
Internal neural variability can induce drift of memory ensembles through synaptic plasticity, allowing for encoding of temporal information.
- Altera Processor Solutions IP Coreon April 28, 2024 at 5:00 pm
It supports the same features ... The TSN Network Core (Switched End Node) from NetTimeLogic is a standalone Time Sensitive Networking (TSN) core according to IEEE 802.1, IEEE 1588 and IEC 62439-3 ...
- How Neural Concept’s aerodynamic AI is shaping Formula 1on April 14, 2024 at 8:00 am
NCS will then dig into its neural network to suggest improvements or modifications, possible paths in a 3D game of choose-your-own-adventure. The human engineer then picks the most promising ...
- Predicting Lithium-Ion Battery Remaining Useful Life Using SDAE-Transformer Fusion Modelon April 11, 2024 at 6:31 pm
In a paper published in the journal Electronics, researchers introduced a novel fusion model for predicting ... techniques such as recurrent neural networks (RNNs) and autoencoders, show promise ...
- Neural Network Learningon April 5, 2024 at 10:34 pm
Meir, R. and Maiorov, V.E. 2000. On the optimality of neural-network approximation using incremental algorithms. IEEE Transactions on Neural Networks, Vol. 11, Issue ...
- Machine Learning with Neural Networkson February 7, 2024 at 8:12 pm
Korouzhdeh, Tahereh Eskandari-Naddaf, Hamid and Kazemi, Ramin 2021. Hybrid artificial neural network with biogeography-based optimization to assess the role of cement fineness on ecological footprint ...
- neural networkson August 14, 2022 at 8:49 pm
WIRED is where tomorrow is realized. It is the essential source of information and ideas that make sense of a world in constant transformation. The WIRED conversation illuminates how technology is ...
- News tagged with neural networkson August 15, 2020 at 3:18 am
Scientists used a neural network, a type of brain-inspired machine learning algorithm, to sift through large volumes of particle collision data. Particle physicists are tasked with mining this ...
- Neural Networks Control A Toy Caron January 3, 2012 at 1:24 am
We have to admit that he nailed it with his neural network controlled car. There’s not much to the build; it’s just an Android phone, an Arduino and a toy car. The machine learning part of ...
via Google News and Bing News