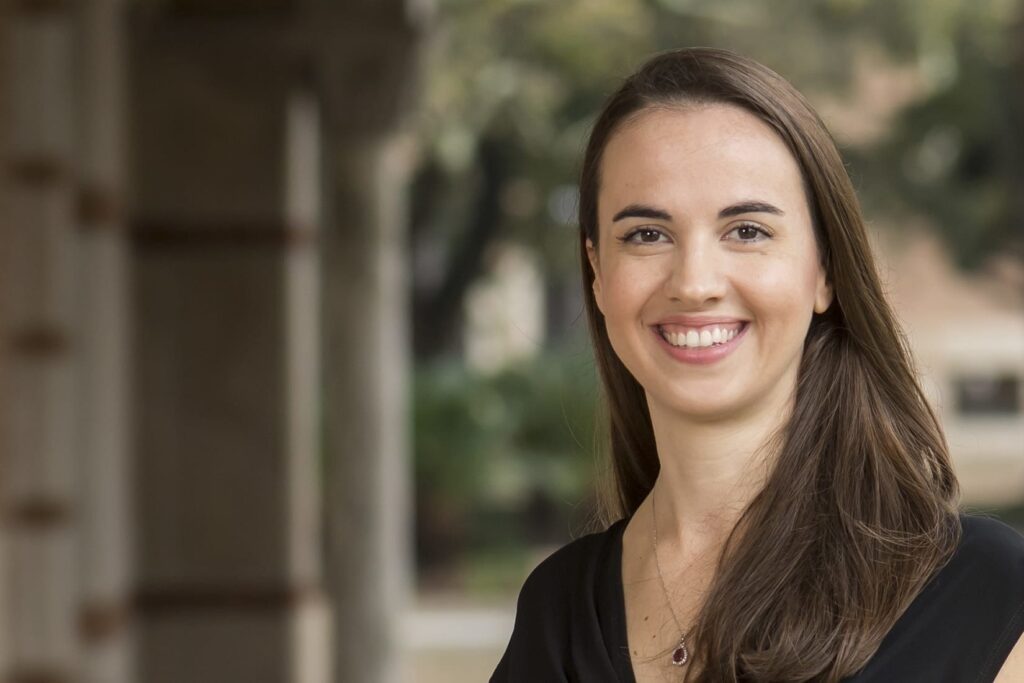
Rice U. expert: Key is creating ML systems that question their own predictions
Rice University statistician Genevera Allen says scientists must keep questioning the accuracy and reproducibility of scientific discoveries made by machine-learning techniques until researchers develop new computational systems that can critique themselves.
Allen, associate professor of statistics, computer science and electrical and computer engineering at Rice and of pediatrics-neurology at Baylor College of Medicine, will address the topic in both a press briefing and a general session today at the 2019 Annual Meeting of the American Association for the Advancement of Science (AAAS).
“The question is, ‘Can we really trust the discoveries that are currently being made using machine-learning techniques applied to large data sets?’” Allen said. “The answer in many situations is probably, ‘Not without checking,’ but work is underway on next-generation machine-learning systems that will assess the uncertainty and reproducibility of their predictions.”
Machine learning (ML) is a branch of statistics and computer science concerned with building computational systems that learn from data rather than following explicit instructions. Allen said much attention in the ML field has focused on developing predictive models that allow ML to make predictions about future data based on its understanding of data it has studied.
“A lot of these techniques are designed to always make a prediction,” she said. “They never come back with ‘I don’t know,’ or ‘I didn’t discover anything,’ because they aren’t made to.”
She said uncorroborated data-driven discoveries from recently published ML studies of cancer data are a good example.
“In precision medicine, it’s important to find groups of patients that have genomically similar profiles so you can develop drug therapies that are targeted to the specific genome for their disease,” Allen said. “People have applied machine learning to genomic data from clinical cohorts to find groups, or clusters, of patients with similar genomic profiles.
“But there are cases where discoveries aren’t reproducible; the clusters discovered in one study are completely different than the clusters found in another,” she said. “Why? Because most machine-learning techniques today always say, ‘I found a group.’ Sometimes, it would be far more useful if they said, ‘I think some of these are really grouped together, but I’m uncertain about these others.’”
Learn more: Can we trust scientific discoveries made using machine learning?
The Latest on: Machine-learning discoveries
[google_news title=”” keyword=”machine-learning discoveries” num_posts=”10″ blurb_length=”0″ show_thumb=”left”]
via Google News
The Latest on: Machine-learning discoveries
- Better identity threat detection sought by new Semperis ML-based toolon May 1, 2024 at 1:57 pm
SiliconAngle reports that more robust high-risk identity threat discovery and response efforts are being aimed by enterprise identity protection startup Semperis with its new machine learning-based ...
- Shiru harnesses AI to launch protein discovery platform: ‘It’s like Amazon for proteins…’on May 1, 2024 at 8:17 am
Shiru is launching a first-of-its-kind discovery platform and marketplace for proteins, enabling companies to identify and test proteins.
- Revealing Protein-Ligand Interactions Using AI Enables Drug Discoveryon April 26, 2024 at 7:34 am
Researchers developed a method to assess the ability of small molecules to bind to hundreds of human proteins using AI and ML.
- Pfizer collab with Austrian research institute leads to new AI models for drug discoveryon April 26, 2024 at 3:29 am
A three-year collaboration between Pfizer and the Research Center for Molecular Medicine of the Austrian Academy of Sciences (CeMM), has resulted in a new AI-driven drug discovery method that could ...
- New AI drug discovery powerhouse Xaira rises with $1B in fundingon April 24, 2024 at 3:00 am
If you asked an AI to generate a brand-new drug discovery biotech with a team of some of the biggest names in the biz for you, it couldn’t dream up one like Xaira Therapeutics. | If you asked an AI to ...
- BigHat Biosciences Enters Into Strategic Collaboration to Leverage Machine Learning in Antibody Discovery & Designon April 24, 2024 at 1:00 am
SAN MATEO, Calif.--(BUSINESS WIRE)--BigHat Biosciences, a biotechnology company with an artificial intelligence/machine learning-guided antibody discovery and development platform announced a ...
- Artificial Intelligence and Machine Learning – Webinars and Online Eventson April 18, 2024 at 6:27 pm
Any application of machine learning technologies with the Diomni™ software package ... Focus will be given to the latest advances in small molecule drug discovery and development, biopharma and ...
- AI Identifies New Potential Treatments For Parkinson’s Diseaseon April 18, 2024 at 7:02 am
A new artificial intelligence (AI) based strategy has significantly sped up the identification of potential new drugs to treat Parkinson's disease. The work, published in the journal Nature ...
via Bing News