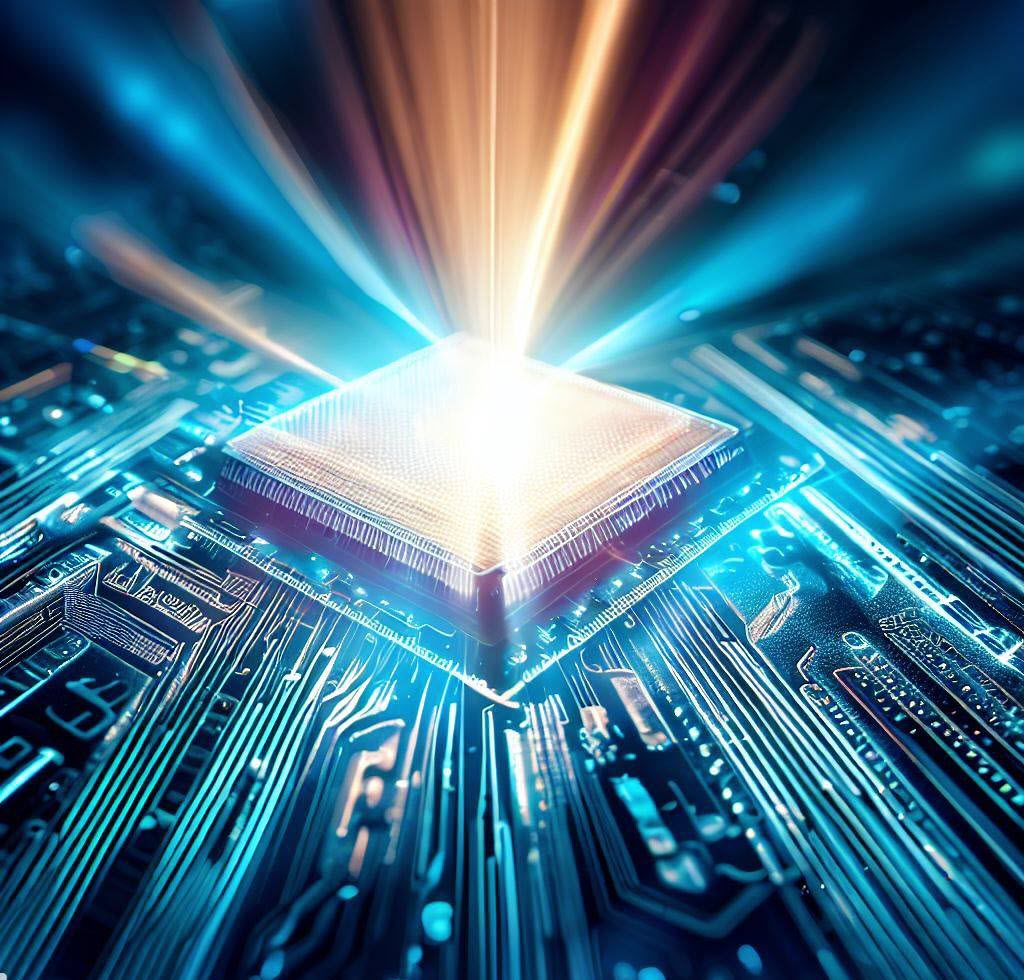
via AI.Nony.Mous
In a groundbreaking and game-changing study that could revolutionize the field of artificial intelligence, researchers have successfully demonstrated that photonic chips can be utilized to train neural networks using on-chip backpropagation—the most widely used method for training these complex systems.
This astonishing breakthrough paves the way for the development of futuristic, optically driven, and energy-efficient machine learning technologies, which hold the potential to dramatically reduce the carbon footprint and costs associated with AI computation.
Neural networks, an approach to machine learning conceptually inspired by the miraculous biology of the human brain, have emerged as the cornerstone of many cutting-edge scientific and commercial AI technologies, including the much-talked-about ChatGPT architectures. As neural networks continue to make significant strides and become increasingly ubiquitous, the energy required to power these awe-inspiring technologies is expected to skyrocket, perhaps doubling every 5-6 months, as some staggering estimates suggest.
Faced with such rapidly increasing energy demands, the quest for more energy-efficient hardware solutions, such as photonic neural networks, has become a critical priority for researchers worldwide. A crucial step towards effectively integrating photonic circuits into neural network applications is the development of a photonic implementation for the so-called backpropagation, the gold standard in neural network training methods.
In a monumental leap forward, Sunil Pai and colleagues have designed and brought to life a cutting-edge hybrid photonic neural network (PNN) chip, capable of performing lightning-fast and efficient on-chip backpropagation training. Employing their state-of-the-art, multilayer photonic integrated circuit, Pai et al. conducted in situ backpropagation training by sending light-encoded errors backwards through the photonic neural network and measuring the optical interference with the original forward-going “inference” signal.
In a series of meticulously designed proof-of-principle experiments, the authors discovered that the PNN performed on par with digital neural network platforms, heralding a new era for scalable, energy-efficient on-chip machine learning. Charles Roques-Carmes, in a thought-provoking and insightful perspective, writes, “Photonic networks are now becoming competitive with state-of-the-art digital platforms, in terms of speed and energy efficiency.”
With this groundbreaking study, it is now anticipated that, in just a few years, large-scale hybrid and all-optical photonic chips will challenge their electronic counterparts in the realm of inference and learning of real-world AI tasks. This pioneering research has the potential to transform the landscape of artificial intelligence, opening up new possibilities for more sustainable and efficient AI technologies that will shape the future of our world.
Original Article: Backpropagation training achieved in photonic neural network
More from: Polytechnic University of Milan
The Latest Updates from Bing News
Go deeper with Bing News on:
On-chip backpropagation
- In Our Opinion: Chip factories will be vital to NY and the country
Of all the resources the nations of the world are vying for, few are more essential to daily life now and in the future than computer chips.
- 3 Blue-Chip Dividend Stocks to Buy That Trade at a P/E of Less than 10
I continue to be optimistic on the upside potential for quality growth stocks. However, for the next few quarters, I am inclined to increase the weight of blue-chip stocks in the portfolio. The key ...
- 7 Blue-Chip Stocks to Buy on the Dip: April 2024
Recent market volatility may have many market participants buying major tech stocks on the dip, but the genuine opportunity may lie with blue-chip stocks to buy on the dip. Blue chips can be a great ...
- Impinj Stock Soars As Tracking-Chip Maker Delivers Beat-And-Raise Report
Internet-of-Things chipmaker Impinj late Wednesday topped Wall Street's targets for the first quarter and crushed views with its outlook for the second quarter. The news sent PI stock soaring in ...
- 1 Top Chip Stock Goes on an AI Acquisition Hunt: Time to Buy?
Shares of Microchip (NASDAQ: MCHP) remain near all-time highs even though its primary customers in the automotive and industrial markets are in the midst of a slump. Clearly, investors are looking ...
Go deeper with Bing News on:
Photonic neural networks
- FORVIA HELLA Shareholders Approve Dividend Proposal at Annual General Meeting
FORVIA HELLA GmbH & Co. KGaA, in its Annual General Meeting held on April 26, 2024, saw its shareholders overwhelmingly approve the proposed ...
- Chip Industry Week In Review
U.S. workforce hubs; Samsung's V-NAND chip; TSMC's A16 process and SoW; NVIDIA's AI acquisitions; IBM to acquire HashiCorp; China/Japan AI EV partnerships; MIT's secure digital IMC chip; a foundry ...
- Demonstration of heralded three-photon entanglement on a photonic chip
Photonic quantum computers are computational tools that leverage quantum physics and utilize particles of light (i.e., photons) as units of information processing. These computers could eventually ...
- AI Efficiency Breakthrough: How Sound Waves Are Revolutionizing Optical Neural Networks
Researchers have developed a way to use sound waves in optical neural networks, enhancing their ability to process data with high speed and energy efficiency. Optical neural networks may provide the ...
- Research Bits: April 23
Alongside the high parallelism and high connectivity of wave optics, the chiplet explores a general and iterative encoding-embedding-decoding photonic computing to effectively increase the scale of ...