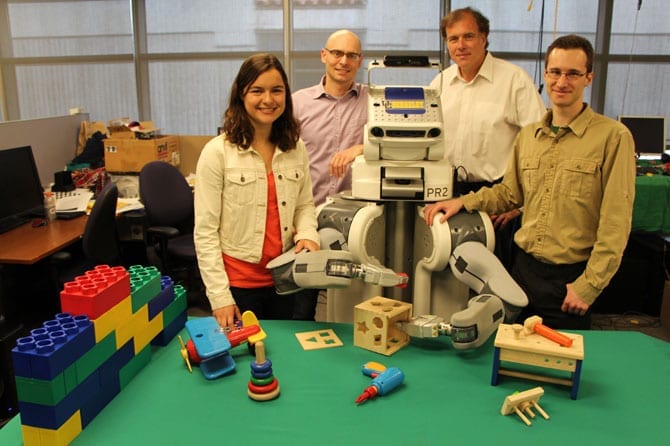
UC Berkeley researchers have developed algorithms that enable robots to learn motor tasks through trial and error using a process that more closely approximates the way humans learn, marking a major milestone in the field of artificial intelligence.
They demonstrated their technique, a type of reinforcement learning, by having a robot complete various tasks — putting a clothes hanger on a rack, assembling a toy plane, screwing a cap on a water bottle, and more — without pre-programmed details about its surroundings.
“What we’re reporting on here is a new approach to empowering a robot to learn,” said Professor Pieter Abbeel of UC Berkeley’s Department of Electrical Engineering and Computer Sciences. “The key is that when a robot is faced with something new, we won’t have to reprogram it. The exact same software, which encodes how the robot can learn, was used to allow the robot to learn all the different tasks we gave it.”
The latest developments will be presented on Thursday, May 28, in Seattle at the International Conference on Robotics and Automation (ICRA). Abbeel is leading the project with fellow UC Berkeley faculty member Trevor Darrell, director of the Berkeley Vision and Learning Center. Other members of the research team are postdoctoral researcher Sergey Levine and Ph.D. student Chelsea Finn.
The work is part of a new People and Robots Initiative at UC’s Center for Information Technology Research in the Interest of Society (CITRIS). The new multi-campus, multidisciplinary research initiative seeks to keep the dizzying advances in artificial intelligence, robotics and automation aligned to human needs.
“Most robotic applications are in controlled environments where objects are in predictable positions,” said Darrell. “The challenge of putting robots into real-life settings, like homes or offices, is that those environments are constantly changing. The robot must be able to perceive and adapt to its surroundings.”
Neural inspiration
Conventional, but impractical, approaches to helping a robot make its way through a 3D world include pre-programming it to handle the vast range of possible scenarios or creating simulated environments within which the robot operates.
Instead, the UC Berkeley researchers turned to a new branch of artificial intelligence known as deep learning, which is loosely inspired by the neural circuitry of the human brain when it perceives and interacts with the world.
“For all our versatility, humans are not born with a repertoire of behaviors that can be deployed like a Swiss army knife, and we do not need to be programmed,” said Levine. “Instead, we learn new skills over the course of our life from experience and from other humans. This learning process is so deeply rooted in our nervous system, that we cannot even communicate to another person precisely how the resulting skill should be executed. We can at best hope to offer pointers and guidance as they learn it on their own.”
In the world of artificial intelligence, deep learning programs create “neural nets” in which layers of artificial neurons process overlapping raw sensory data, whether it be sound waves or image pixels. This helps the robot recognize patterns and categories among the data it is receiving. People who use Siri on their iPhones, Google’s speech-to-text program or Google Street View might already have benefited from the significant advances deep learning has provided in speech and vision recognition.
Applying deep reinforcement learning to motor tasks has been far more challenging, however, since the task goes beyond the passive recognition of images and sounds.
“Moving about in an unstructured 3D environment is a whole different ballgame,” said Finn. “There are no labeled directions, no examples of how to solve the problem in advance. There are no examples of the correct solution like one would have in speech and vision recognition programs.”
Read more: New ‘deep learning’ technique enables robot mastery of skills via trial and error
The Latest on: Deep Learning
[google_news title=”” keyword=”Deep Learning” num_posts=”10″ blurb_length=”0″ show_thumb=”left”]
via Google News
The Latest on: Deep Learning
- Study: Deep learning-based whole-body PSMA PET/CT attenuation correction utilizing Pix-2-Pix GANon May 9, 2024 at 2:12 pm
A new research paper was published in Oncotarget, titled "Deep learning-based whole-body PSMA PET/CT attenuation correction utilizing Pix-2-Pix GAN." ...
- Deep learning empowers reconfigurable intelligent surfaces in terahertz communicationon May 9, 2024 at 10:39 am
The escalating demand for wireless data traffic, driven by the proliferation of internet-of-things devices and broadband multimedia applications, has intensified the search for innovative solutions in ...
- Ensemble deep learning models enhance early diagnosis of Alzheimer's disease using neuroimaging dataon May 9, 2024 at 7:24 am
By Dr. Priyom Bose, Ph.D. A recent Nature Mental Health study assessed the developments in ensemble deep learning (EDL) models used to characterize and estimate AD. Study: Ensemble deep learning for ...
- Can We Have Meaning As Well As Fun? Nick Bostrom’s “Deep Utopia”on May 9, 2024 at 6:30 am
Review of Nick Bostrom’s new book, “Deep Utopia”. The possibility of meaning in a technologically mature world ...
- Machine learning tool flags undiagnosed immunodeficiency diseaseon May 9, 2024 at 6:00 am
A machine learning model can effectively sift through EHR data to rank patients' likelihood of having common variable immunodeficiency disease.
- Road to safer self-driving cars is paved with deep learningon May 8, 2024 at 10:34 pm
Breakthrough in understanding confidence levels in deep learning AI has implications for fields ranging from transportation to healthcare.
- ARVO 2024: How a deep learning model can benefit femtosecond laser-assisted cataract surgeryon May 7, 2024 at 1:02 pm
Dustin Morley, PhD, principal research scientist at LENSAR, discusses research on applying deep learning to benefit FLACS procedures.
- Carnegie Mellon develops deep-learning alternative to in-situ PBF-LB monitoringon May 7, 2024 at 5:26 am
Researchers have developed a deep-learning approach to capture melt pools in PBF-LB Additive Manufacturing using airborne or thermal emissions ...
- AI Deep Learning Improves Brain-Computer Interface Performanceon May 6, 2024 at 8:54 am
AI deep learning powers a brain-computer interface that enables humans to continuously control a cursor using thoughts.
- Deep learning techniques for Hyperspectral image analysis in agricultureon May 5, 2024 at 5:20 am
These innovations underline the industry’s brisk evolution, paving the way for hyperspectral imaging (HSI) and deep learning to further revolutionize farming practices, enhancing efficiency and ...
via Bing News