Researchers developed a versatile remote gaming interface that allowed external experts as well as hundreds of citizen scientists all over the world through multiplayer collaboration and in real time to optimize a quantum gas experiment in a lab at Aarhus University.
Surprisingly, both teams quickly used the interface to dramatically improve upon the previous best solutions established after months of careful experimental optimization. Comparing domain experts, algorithms and citizen scientists is a first step towards unravelling how humans solve complex, natural science problems.
In a future characterized by algorithms with ever increasing computational power, it becomes essential to understand the difference between human and machine intelligence. This will enable the development of hybrid-intelligence interfaces that optimally exploit the best of both worlds. By making complex research challenges available for contribution by the general public, citizen science does exactly this. Numerous citizen science projects have shown that humans can compete with state-of-the-art algorithms in terms of solving complex, natural science problems.
However, these projects have so far not addressed why a collective of citizen scientists can solve such complex problems. An interdisciplinary team of researchers from Aarhus University, Ulm University, and the University of Sussex, Brighton have now taken important first steps in this direction by analyzing the performance and search strategy of both a state-of-the-art computer algorithm and citizen scientists in their real-time optimization of an experimental laboratory setting.
The Alice Challenge
In the ‘Alice Challenge’, Robert Heck and colleagues gave both experts and citizen scientists live access to their ultra-cold quantum gas experiment. This was made possible by using a novel remote interface created by the team at ScienceAtHome, Aarhus University. By manipulating laser beams and magnetic fields, the task was to cool as many atoms as possible down to extremely cold temperatures just above absolute zero at -273.15°C. This so-called Bose-Einstein condensate (BEC) is a distinct state of matter (like solid, liquid, gas or plasma) that constitutes an ideal candidate for performing e.g. quantum simulation experiments and high precision measurements.
As detailed below both groups successfully used the remote interface to improve on previously optimal solutions. In this first ever citizen science experimental optimization challenge with real-time feedback the researchers further quantified the behavior of citizen scientists. They concluded that what makes human problem solving unique is how a collective of individuals balance innovative attempts and refine existing solutions based on their previous performance.
Quantum optimization as a remote service
Quantum technology is increasingly stepping out of university labs and into the corporate world. For high-performance and robust applications exceptional levels of control of the complex systems is needed and new methodologies in both theoretical and experimental science are needed.
This requires interdisciplinary and often trans-institutional collaborations, which in turn necessitates the development of efficient interfaces to allow each of the experts to contribute as efficiently as possible.
In recent years, remote interfaces to experimental apparatuses have started to appear, however, they are always focused either on educational settings or the investigation of a very particular experimental setup.
In contrast, Robert Heck and colleagues in the current work set out to develop a flexible remote interface and a powerful optimization algorithm that can potentially be applied to many other settings in the future. The experimental production of BECs serves as an ideal test-bench:
The RedCRAB package
“Making machines play the Alice Challenge alongside with humans over the internet required us to create a new software package, RedCRAB, for remote optimization of quantum experiments,” explained Tommaso Calarco (Forschungszentrum Jülich/University of Cologne) and Simone Montangero (Padova University), leaders of the Ulm optimization team.
RedCRAB is ideally suited for problems with many control parameters where the exact theoretical modelling of the system is unknown and other traditional optimization methods fail. It furthermore has the advantage that the optimization experts can easily adjust algorithmic parameters and exploit its full potential without requiring intermediate communication with the experimental team. Moreover, the efficiency of the optimization can be analyzed, and based on that, algorithmic improvements can be made and easily transferred to future experiments.
Tommaso Calarco is extremely excited about the results:
“RedCRAB optimization worked so well that it is now applied in several labs around the world to enhance the performance of quantum devices. We plan to extend this as a cloud service that we believe will likely result in faster development of the theoretical understanding, of the algorithmic development and overall of quantum science and technologies.”
Can any research challenge be turned into a game?
As mentioned above, game interfaces have in recent years enabled non-experts to use their creativity and intuition to contribute to various scientific fields. In 2016, the Aarhus research group reported the results of the first quantum citizen science game, Quantum Moves, in Nature. In the game, the players contributed to finding fast and efficient solutions to atomic transport in a quantum computing architecture.
The clear water-like analogy of that particular game and the scarcity of other quantum games has since sparked the criticism that perhaps non-experts can only contribute in research topics for which a clear classical analogy can be established. Since this can rarely be established for any given research challenge, it could seem that the gamification approach is of very limited general applicability and Quantum Moves was just a very special case.
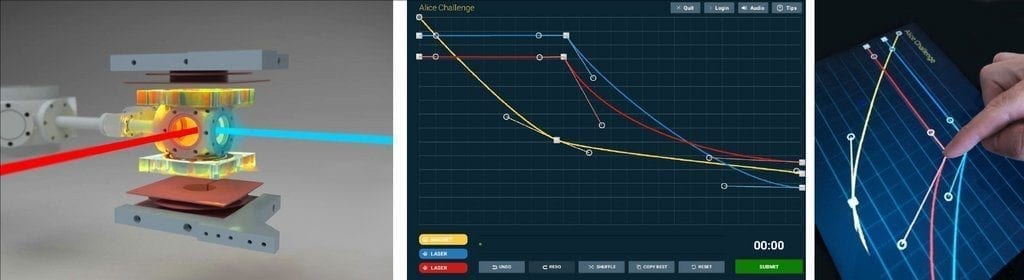
To test this hypothesis, the remote interface to the ultra-cold atoms experiment in Aarhus was turned into a citizen science game, the Alice Challenge. Concretely, the players controlled laser intensities and magnetic fields of the experimental sequence. As illustrated in the figure the “game” interface is far from intuitive and perhaps not very entertaining. The players drag one or more of the curves, push the submit button. Then the solution was sent to the lab, the experiment conducted, and roughly 35 seconds later the result is communicated to the player.
Two weeks of gaming – and better solutions
Robert Heck, one of the lead scientists in designing the Alice Challenge and first author of the paper:
“This realizes the first ever citizen science experimental optimization challenge with real-time feedback in any field. In the Alice Challenge 600 citizen scientists had over two weeks access to our lab in Aarhus. Within this time, 7577 solutions were submitted and realized in the lab. It was also a challenge for us. As our participants came from all over the world we had to keep the experiment online for two weeks straight without interruptions.”
Although the players did not have any formal training in experimental physics they still consistently managed to find surprisingly good solutions. Why? One hint came from an interview with a top-player, a retired Italian microwave system engineer. He said, that for him participating in the Alice Challenge reminded him a lot of his previous job as an engineer. He never attained a detailed understanding of microwave systems but instead spent years developing an intuition of how to optimize the performance of his “black-box”.
“This is extremely exciting. We humans may develop general optimization skills in our everyday work life that we can efficiently transfer to new settings. If this is true, any research challenge can in fact be turned into a citizen science game,” said Jacob Sherson, head of the ScienceAtHome project.
Are citizen scientists really better?
How can untrained amateurs using an unintuitive game interface outcompete expert experimentalists? One answer may lie in an old Herbert Simon quote: “Solving a problem simply means representing it so as to make the solution transparent”. In this view, the players may be performing better, not because they have superior skills but because the interface they are using makes another kind of exploration “the obvious thing to try out” compared to the traditional experimental control interface.
“The process of developing fun interfaces that allow experts and citizen scientists alike to view the complex research problems from different angles, may contain the key to developing future hybrid intelligence systems in which we make optimal use of human creativity,” explained Jacob Sherson.
Social science in the wild
Another reason for the success of the citizen scientists is likely due to the multiplayer collaboration that the remote interface facilitated. Testing this hypothesis involved a substantial advancement compared to traditional social science.
Carsten Bergenholtz and Oana Vuculescu, the social science experts of the project:
“In the social sciences we are interested in how people solve problems. However, we often invite them into a social science lab to solve artificial problems, which are not directly connected to the real world. Furthermore, individuals in the lab usually solve these artificial problems alone. In contrast, our Alice Challenge was a unique opportunity to do social sciences ‘in the wild’, i.e. players solved a real problem and we allowed players to collaborate and learn from each other. Overall, this enables us to address why a collective of citizen scientists are surprisingly good at solving such complex problems.”
The researchers find that individuals at the top or bottom of the leaderboard behave differently. Well-performing players engage in small changes to their proposed solutions whereas poorly-performing players explore the unknown and apply larger changes. As poorly performing players move upwards in the ranks and, vice versa, well-performing players move downwards individuals adapt their search accordingly.
On a collective level, this means there are always some players at the top of a leaderboard that optimize the current best solution as well as some players at the bottom of a leaderboard that innovate and try out completely new solutions. This was directly compared to the behavior of the RedCRAB algorithm that was much more local in nature – focusing on small steps to iteratively improve the current solution instead of broadly searching the overall landscape.
A unique insight
“These findings provide insight into the unique human ability to collectively solve complex problems. Leveraging this knowledge will allow for the design of hybrid intelligence interfaces that combine the computational strengths of AI with the advantages of human intuition,” said Carsten Bergenholtz and Oana Vuculescu.
Mark Bason at University of Sussex is looking into the future:
“Progress in science is very frequently the result of close collaboration between established groups, such as those from academia or industry. However, technology has advanced so far that many new interactions are possible. By opening up our research, we can now benefit from the skills of players, algorithms and hybrid approaches of the two.”
The Latest on: Citizen science
[google_news title=”” keyword=”citizen science” num_posts=”10″ blurb_length=”0″ show_thumb=”left”]
via Google News
The Latest on: Citizen science
- Citizen science: Dept. of Conservation, Agriculture ask for help with black vulture studyon April 26, 2024 at 1:01 pm
MDC, USDA needs public’s help with black vulture studyThe Missouri Department of Conservation (MDC) is partnering with the U.S. Department of Agriculture (USDA) ...
- Exploding stars send out powerful bursts of energy − I'm leading a citizen scientist project to classify and learn about these bright flasheson April 26, 2024 at 11:00 am
Swift is a multiwavelength space telescope that scientists are using to find out more about these mysterious gamma-ray flashes from the universe.
- Across the Finish Line! Citizen Science Month Final Days.on April 26, 2024 at 10:42 am
One Million Acts of Science challenge met, but lots of important project work is still left!
- You might find a rare species in your backyard: How global citizen science contributes to biodiversity knowledgeon April 26, 2024 at 9:28 am
While it can be hard for us to notice as we go about our busy lives, cities are filled with indigenous plants, fungi, insects, spiders and other little creatures, as well as birds, frogs and reptiles.
- 'Citizen scientists' help NASA discover over 1,000 new asteroids using Hubble Telescopeon April 25, 2024 at 5:06 pm
NASA said volunteers scanned 19 years' worth of images from the Hubble Telescope, using a new approach that could lead to other discoveries.
- Gotham Whale, a New York City-based nonprofit, allows citizen scientists to assist in conservation effortson April 25, 2024 at 6:41 am
A whale-watching excursion that embarks straight from New York City offers passengers an opportunity to and assist in much-needed conservation efforts.
- Sewage-free rivers a step closer after citizen science group's official launchon April 23, 2024 at 5:00 pm
NORTHWICH’S rivers are a step closer to being sewage free. Saturday, April 20, saw the official launch of citizen science water quality testing group, Northwich River Heroes, at Grozone Community ...
- ‘Not the only birder in the village’: how citizen science is the bedrock of tackling species losson April 20, 2024 at 3:59 pm
Birdwatching may have started out as a hobby, but active volunteers are helping bridge data gaps of threatened species and reaping real world outcomes as they go ...
- Citizen Science Month and #OneMillionActsOfScience Needs You!on April 19, 2024 at 3:00 pm
It's a packed week, with Earth Day, Arbor Day, The City Nature Challenge and more!
via Bing News